Let
{S1, ..., Sn} be an arrangement of
n linear subspaces of dimensions
{d1, ..., dn} embedded in
D dimensional space. Consider a given collection of
N = Σi Ni data points
{y1, ..., yN} drawn
from the
n subspaces.
The sparse subspace clustering (SSC) algorithm [1], addresses the subspace clustering problem using
techniques from sparse representation theory. This algorithm is based on the observation that each data point
y ∈ Si can
always be written as a linear combination of all the other data points in
{S1, ..., Sn}. However, generically, the
sparsest
representation is obtained when the point
y is written as a linear combination of points in its own subspace. A representation that uses other points from its own subspace is called
subspace-preserving. In [1] we show that when the subspaces are low-dimensional and
independent (dimension of the sum is equal to the sum of dimensions), subspace-preserving representation can be obtained by using
L1 minimization.
The segmentation of the data is found by applying spectral clustering to a similarity graph formed using the sparse coefficients. More specifically, the SSC algorithm proceeds as follows.
Algorithm 1
Input: A set of points
{y1, ..., yN} lying in a union of
n subspaces
{S1, ..., Sn}.
1: Solve the sparse optimization problem
minC ||C||1 s.t. Y = YC, diag(C) = 0, where
C = [c1, ..., cN] is the matrix whose
i-th column corresponds to the sparse representation of
yi using
Y = [y1, ..., yN].
2: Normalize the columns of
C as
ci ← ci / ||ci||∞.
3: Form a similarity graph with
N nodes representing the data points. Set the weights on the edges between the nodes by
W = |C| + |C|T.
4: Apply spectral clustering to the similarity graph.
Output: Segmentation of the data.
An example of data drawn from three subspaces and the corresponding adjacency matrix and similarity graph is shown in the figure below. For each data point, the
L1
minimization gives subspace-preserving representation, hence the adjacency matrix has a block-diagonal structure and the similarity graph has three connected components. Thus, using standard
spectral clustering methods, we can recover the components of the similarity graph, hence the segmentation of the data.
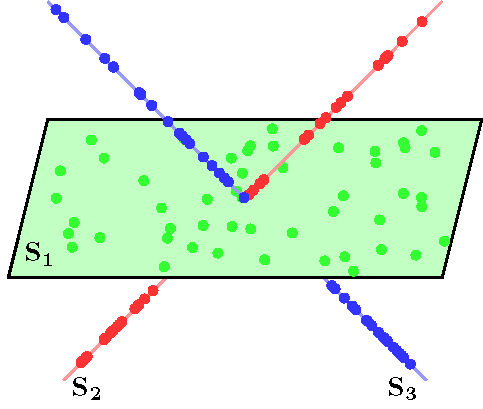 |
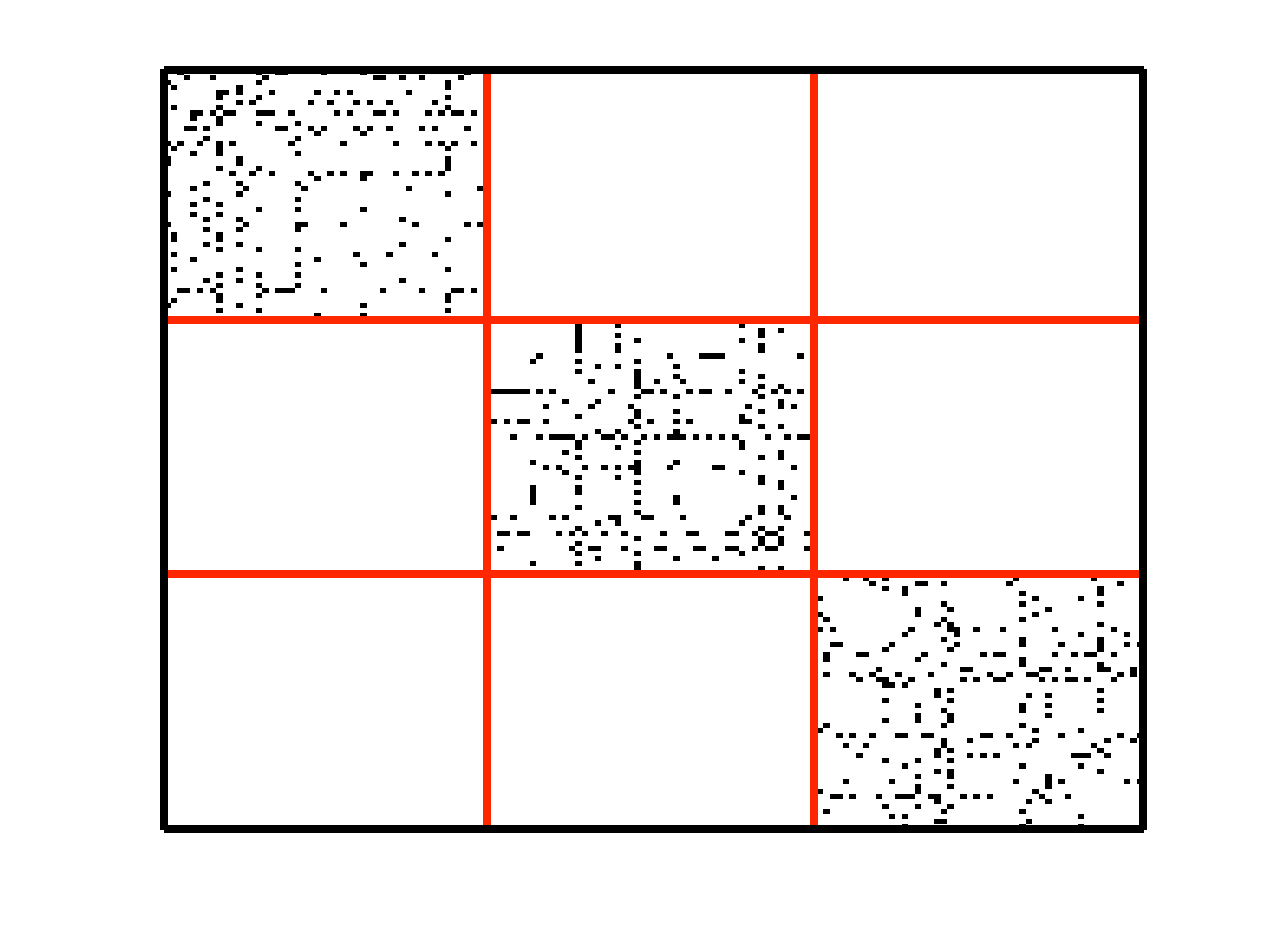 |
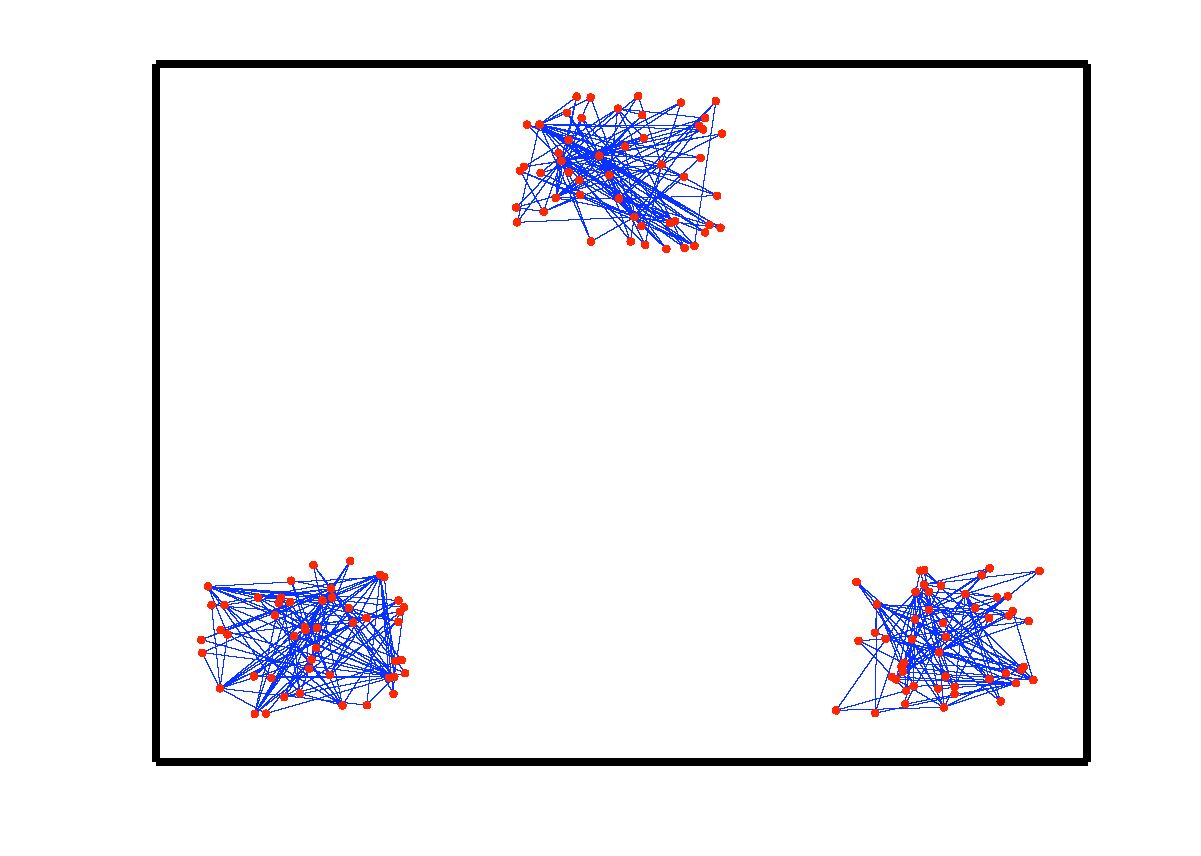 |
Data drwan from 3 subspaces |
Matrix of sparse coefficients |
Similarity graph |
However, requiring the subspaces to be independent is a strong assumption in practice. In [2], we address the more general problem of clustering
disjoint subspaces
(each pair of subspaces intersect only at the origin). We show that under certain
conditions relating the principal angles between the subspaces and the distribution of the data points across all the subspaces, subspace-preserving representation can still be found by using convex
L1 optimization. This result represents a significant generalization
with respect to the sparse recovery literature, which addresses the sparse recovery of signals in a
single subspace. The subspace
clustering problem is also much more challenging than block-sparse recovery problem, whose goal is to write a signal as
a linear combination of a small number of known subspace bases. First, we do not know the basis for any of the subspaces nor do we know
which data points belong to which subspace. Second, we do not have any restriction on the number of subspaces, while existing methods
require this number to be large.